the future
interactions
Our Mission
We fuse our artificial intelligence and cryptography expertise to engineer solutions that radically improve web3 accessibility and scalability.
matters?
Web3 must still be easier to use, and its capabilities must be expanded. Seamless, secure interaction is the key to catalyzing mass adoption.
Semiotic Labs is the R&D core developer team in The Graph protocol. We're not just developers but innovators with a proven track record. Having incubated Odos – a leading DeFi liquidity aggregator and trading platform – we aim to continue building beautiful, impactful products for crypto and web3.
Join our team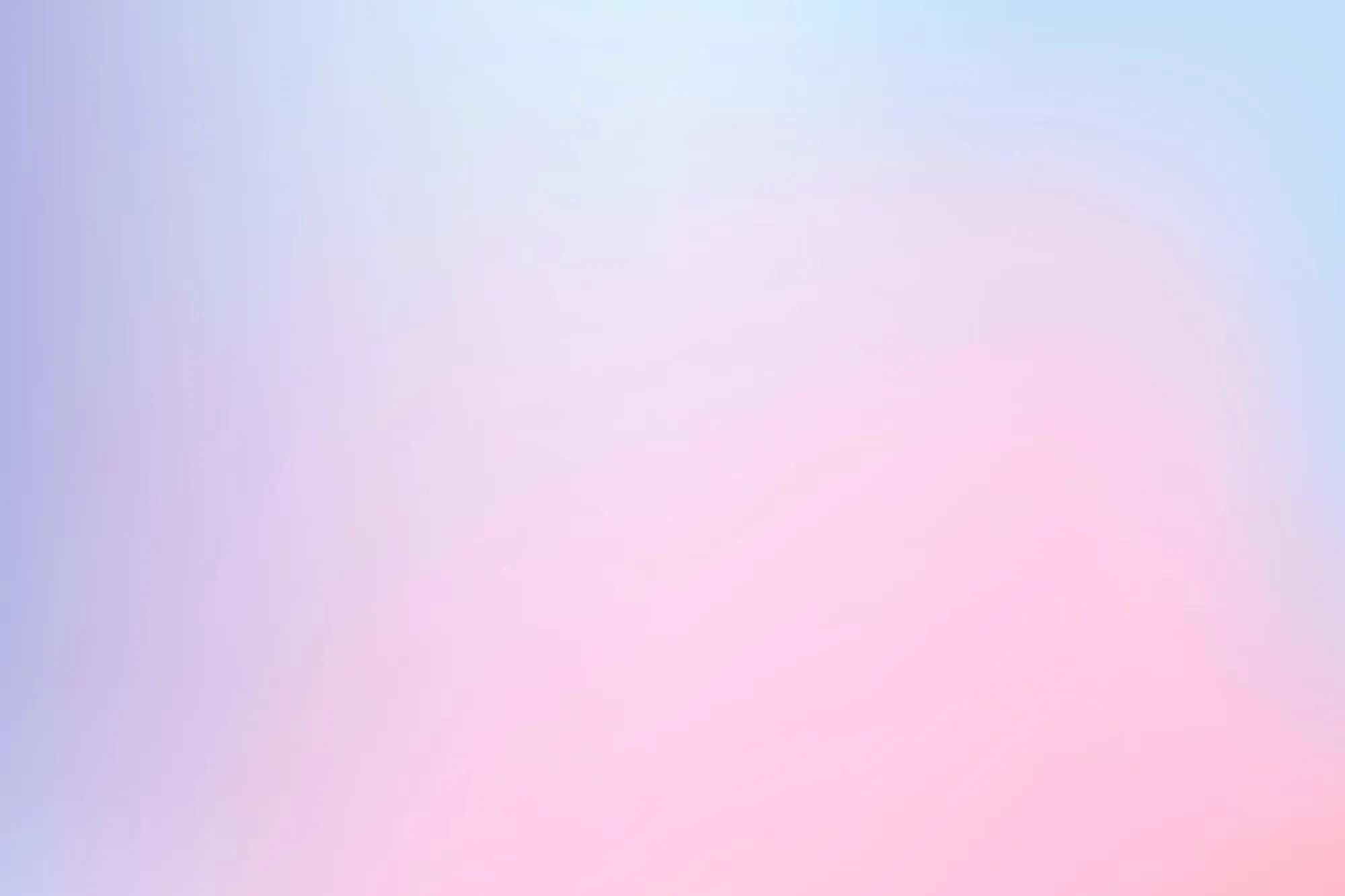
What we
built
Through meticulous effort and unwavering commitment, we craft innovative products that are a testament to our vision.
See our products